About T-test:
I have thought a proper way to make the t-test accessible; but, I figured out that it is not helpful to skip z-score and z-test. Before I tell any further, I'd like to mention that the t-test and z-test are virtually the same. Some differences are discussed later.
I will talk about z-test first.
I am repeating things because statistics tend to confuse us. So, let's start with standard error.
Do you remember the concept of the standard error of the mean? I have told several times that it is important -- it pops out here. The standard error of the mean refers to the standard deviation of the means of (many imaginary) samples from a particular population you are interested in.
The concept, the standard error of the mean gives the "possible range of mean scores" of samples (given a size of N) from a population. After you figure out the range of possible mean scores, you can estimate the mean score of the population. For example, in the IQ score test, suppose it is known that the national IQ score average is 100 (from the reliable statistical source) and the standard deviation is 10. With this information, you can calculate the standard error of the means with the formula (see the figure for the formula). Since the formula wants you to provide the number of the sample (sample size), you decided that you will take 25 individuals for a sample. Then, the standard error of the means of IQ score test, with the sample size 25, would be 2.
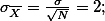
Also we know that the mean of (the samples means) is the same as the population mean. That is,

.
Because the standard error is actually the standard deviation of sample means, we can use the 68-95-99 rule here to estimate the range of the possible population mean.
1 standard error unit: 1*se = 2
2 standard error units: 2*se = 4
3 standard error units: 3*se = 6
Mean +- 1*se = 100 +- 1(2) = 98 - 102 with 68% confidence;
Mean +- 2*se = 100 +- 2(2) = 96 - 104 with 95% confidence;
Mean +- 3*se = 100 +- 3(2) = 94 - 106 with 99% confidence
What it tells is that if we take a random sample (not imaginary, n=25), the mean should be found in the above ranges. With 68% sure, the mean of the sample would lie between 98 to 102. With 95% sure, the mean of the sample would lie between 96-104. With 99% sure, the mean of the sample would be found between 94-106.
Now, suppose that you took a sample (n=25) and found that the mean score for this sample was 105. From this number, you can immediately assume that there is something wrong. That is, with 95% confidence, the means of samples (n=25) should fall between the 96 and 104. But, the mean of this particular sample is 105. So, we may think that this particular sample is unusual in comparison to the characteristic of the population. Or, we can think another way around -- what we were told as national average IQ score (=100) may be wrong.
Stop here. This is a recap for the concept of the standard error of the means.
In the above, we figured out the ranges first, got the mean of a sample; and compare it to the ranges. We can simplify these by using z-score. In other words, we can regard the numerical value of one standard error as one unit. In this case, because the standard error is 2, one unit of standard error is 2. How far the mean of the particular sample (mean = 105) was off from the mean of the population in the above example? -- 5 (105 (mean of the sample) - 100 (mean of the population)). Numerically it is off by 5. Now, how many standard error units is this mean (105) off from the mean of the population? Since we regard numerical value 2 as one unit of standard error, it is 2.5 units off from the population (5 divided by the unit of standard error, 2). This sounds complex. But, if we see the mathematical form below:

.
As we figured out the result is 2.5. This value is called z-value (usually z means standardized). If you take a look at the below:
1 standard error unit: 1*se = 2 -- 68% -- z-score = 1
2 standard error units: 2*se = 4 -- 95% -- z-score = 2
3 standard error units: 3*se = 6 -- 99% -- z-score = 3
In stead of dealing with 2, 4, and 6 which are numerical, now we can use 1, 2, and 3 to compare. In the example, we got 2.5 (z-score) which is bigger than the underlined 2. Therefore, we can see that the mean of the sample (105) falls out of the range provided with 95% certainty (2 standard error unit). Therefore, we can reach the same conclusion as we did. That is, we may say that, with 95% certainty, this particular sample seems an unusual case considering the given characteristic of the population. Or, we can think another way around -- what we were told as national average IQ score (=100) may be wrong with 95% certainty.
The benefit of using z-score is that now we can compare the obtained value for our sample to the number 2 (if you are required to employ 95% certainty. If you are required to employ 68% certainty, you can use the number 1, right?). This is because we "unit-ized" or "standardized" the score. This was obtained through just dividing the difference (between mean of the sample and the mean of the population) with the standard error of the means.
There is another application of this kind of test. In the above example, we just had one sample and were given the parameters for the population (do you remember the terms, parameter and population?). Suppose that we have two samples, instead of one. And we want to see whether two samples are the same kinds. For example, one sample is students who do not use the class web site. And the other is those who use the class site as a study resource. We got the exam result and want to compare them to see (judge, or decide) whether they are different. This is called two-sample z-test.
For this particular test, we use the standard error of the differences of the means. This is a different kind of standard error. Do you remember that I told you that there are many kinds of standard errors? -- the standard error of the probabilities, the standard error of the means, standard error of the differences of the means, etc. There are many others. All stem from the same idea, though. For this term, the standard error of the differences of the means, we do the same imaginary experiment. We take a sample from each group (or population); get the mean of the each sample; compare them (by subtracting one mean from the other); record the difference (compared result); take another sample from each group (or population); get the sample means; compare them; record the difference; ..... You keep doing it and get the statistics out of the record. The standard deviation obtained from this record has the exact same characteristics as the standard error of the means. And this is called the standard error of the differences of the means.
Let's go back to the example. We have 89.5 and 85 as the mean of the each sample -- those who use the class web site and those who do not. Suppose that we got the standard error of the differences of the means (1.5). We can use the same procedure as the above (with some modification: we now subtract one mean from the other)

.
Here, since we have the z-value, 3, which is bigger than 2 (assumed that 95% certainty was employed), we can argue that with 95% certainty, the two sample are different from each other in terms of the performance on the exam.
What can we say about this? We can say that those who use the class web site regularly tends to have higher exam scores in comparison to those not. The difference found in the above samples turned out to be statistically significant. That is, the differences was not due to a mere chance.
Now, about t-test (you have been waiting too long).
But, there is nothing much to say about because it is the almost same thing as the Z-test. Some points that I want to make though are
- that you need to refer to t-distribution table to make the statistical decision (rejecting the null hypothesis, p. 399 in the textbook.); As we discussed, the t-test (z-test) score uses the concept of standard error of mean differences (between two groups). So, our common sense (if it works ok) tells us, "Hey, if the mean difference between two groups are relatively small, the test score will be small. In fact, if they are identical, the test score should be 0 (zero)." So, when you look up the t-test table with your "calculated t-test score (value)", your calculated score should be bigger than the critical score (value) in order to say that there IS difference between the two groups.
- that the T-test is used with small sample sizes;
- the T-test is mostly used with two-sample problems (two-sample t-test; same thing as two-sample z-test).
I will give you an example. Suppose that you are interested in two groups. One is those who attend the review session for the COMM research class. The sample size for this group is 12. The other is those who do not. the sample size for this group is 12. The mean score of the example for each group is 90 and 82 respectively. And the standard error of the differences of the means is given and it is 3. We can use the formula for the T-test, below.

.
You also need to figure out what is the degree of the freedom. The formula for this is:

.
If we take a look at the t-distribution table:
the critical value: 2.074 at 0.05 probability level (p = 0.05).
the critical value: 2.819 at 0.01 probability level (p = 0.001).
Now you need to compare the
calculated t-test value (2.67) and the
critical value found in the table (2.074 at p = 0.05 level and 2.819 at p = 0.001 level). You figure out that the t-test value is bigger than the critical value at 0.05 level. You know that the t-test value depends upon the value of the difference between
bar(X_1) text(and) bar(X_2) (see the above formula) -- if the difference is big the t-test value gets big. If there were no difference between the two groups in the first place, the t-test value is going to be zero (0). So, if obtained t-test value is larger than the critical value, you can say that there seems real difference in exam score between the two groups with 95% confidence. But, it is not the case at the 0.01 level. The obtained t-test value is smaller than the critical value at 0.01 level. Therefore, you cannot say that there seems real difference in the exam score between the two groups with 99% confidence.