This is rather a redudent, long description of chi-square test.
Cross Tabulation (AKA Chi-Square Table or Chi-Square Test)
Before you read this:
- This reading is an option.
- Cross Tabulation is similar to Chi-Square test.
- The document is long because no one seems to
- like to using direct mathematical concepts. There are a lot of repetition
- here (Dr. Rice once talked about this).
- Pictures are taken by your TA at the RUSURE
- campaign directed under Dr. Letterman and Dr. Stewart during the year, 2000.
- The study is ongoing at the SCILS department.
Let's start with what we know first.
- Two variables
- Let's say you are interested in the relationships between the types of religions and opinions about abortion.
You have a hunch that people who have a different religion will have a different opinion about abortion. This actually reveals that you think having a particular religion will affect what to think about abortion. Therefore, particular religions will be the
IndependentVariable (IV). And the opinions abut abortion will be the
DependentVariable (DV).
(Identifying types of variables in your research idea is an important step)
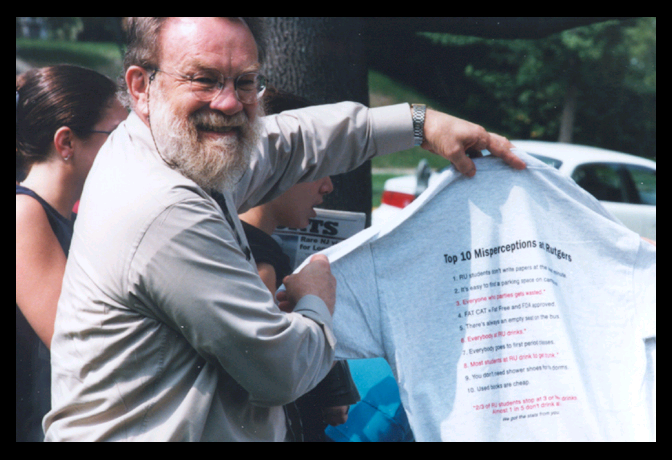
Dean of SCILS at Rutgers promoting RUSURE program [JPG image (603.81 KB)]
We have two variables here. What kinds of values (attributes) do you see for the first variable, types of religions?
Nominal variable. Your initial response would be
Catholic, Protestant, and Judaism. . . . At this point your might not be sure if you thought of all kinds of religions -- yes, certainly, there is Buhdism, too; and many others. Now you use your judgment about this
exhaustivenss problem. With your rationale, you can decide that you make categories as follow: Variable 1: Catholic, Protestant, and Others. The last one,
others, covers everything else, right? So you just escaped from the exhaustivenss problem. As a side note, you may say, "Wait, my religion is not there... and It's unfair!" That's why I said you use your judgment. The rationale for the choice was to see the difference between the two religions and others -- Catholic versus Protestant versus everything else. Or in your gathering samples, you may want to choose only those who are catholic and protestant. Basically, it is your (researcher's) choice. But, as far as sampling method concerned, it should be randomly done.
Now we need to take care of the other variable, the choice of abortion. The values of the variable, choice of abortion, may be rather simple:
Variable 2: Yes, and No.
Hence, this is another nominal variable. At this moment, ask yourself, "Do I feel safe? Have I thought enough?" . . . . If your answer is yes, I might ask you this. By abortion, do you mean to include illegal abortion or just legal abortion? The point is that people might arbitrarily take and answer your question. So, let's decide you focus on legal abortion. The choices are still yes and no.
Please understand that you need "nominal" variables for the chi-square test. More arcurately put, since you decide to pursue the relationships between the two variables (religion and abortion), and figure out that they are nominal variables, you are deciding to use the chi-square test.
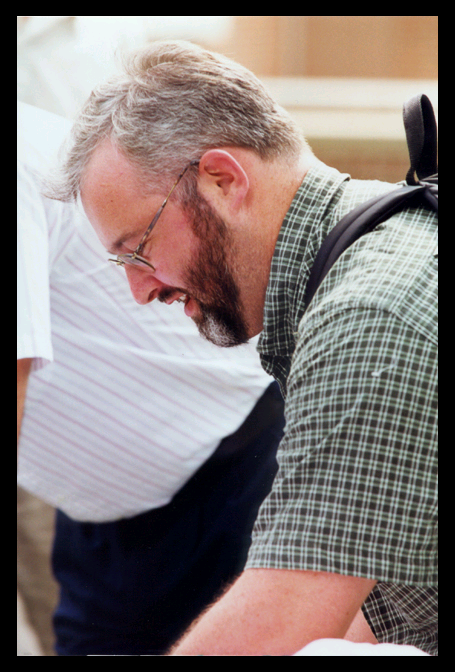
Bill White of SCILS at Rutgers promoting RUSURE program [PNG image (259.91 KB)]
Think about the whole semester at this moment. You generated a research question based on the general ideas (theory) that you earned from your life-time experiences (different religious people will have different opinions about abortion). You defined religion and opinion about abortion in a somewhat implicit way -- That is, you did not clearly defined the terms -- religion and opinion about legal abortion --, but you acted upon the idea that everybody knows what religion is and what is the opinion about abortion; and listed the complete attributes of the variables. This actually covers conceptualization and operationalization. You made a questionnaire based on the above ideas and now are ready to ask people to fill your form out.
- Sample
- The next step is taking a set of sample and ask them to fill the survey questions. I will not discuss this in depth. But, from the lectures, you know there are a lot of issues here -- sampling methods, representativeness of the sample, and so on.
- 2-way contingency table
- The number of sample was 100. In order to organize how the relationships between religions and abortion ideas render, you make a table as follows.
religeon vs abortion opinion |
| Catholic | Protestant | Others | Total |
yes | 5 | 32 | 13 | 50 |
. | | | | |
no | 35 | 10 | 5 | 50 |
. | | | | |
Total | 40 | 42 | 18 | 100 |
Let's forget about the chi-square test for a while and discuss the table itself.
The table is also called frequency table (you counted people) as well as contingency table. Since the table involves two variables, it can be also called bivariate analysis -- bi means two; hey, bicycle! Anyway...
First, think about why you did this. You wanted to compare how people with different religions have different opinions about legal abortion. So, you made a questionnaire and asked 100 people to fill the questions forms out.
From the result, you notice:
- That there were 40 people whose religion is Catholic and
- 42 people whose religion is Protestant. And 18 other people have different religions.
- Among the Catholic, five persons support legal abortion,
- while 35 persons does not.
- Among the Protestant, 32 people are for the abortion issue
- and 10 people are against it.
- 13 persons who have religions other than Catholic and
- Protestant -- no religion was included here, too -- are for legal abortion; and 5 persons
- in the same category are against it.
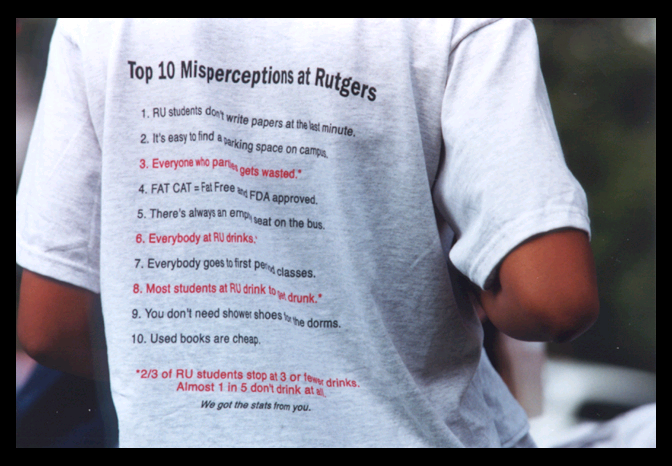
Top 10 misconception about alcohol - RUSURE campaign [JPG image (611.69 KB)]
From this observation, can you say that there are difference in the opinions about legal abortion between or among people who have different religions? In simple words, can you conclude that the Catholic have a different opinion about legal abortion from others? You'd say, "Sure, why not?" You can back up the conclusion by arguing that "only 5 out of the 40 Catholic (12.5%) believe legal abortion can or should be allowed. But, 32 out of the 42 Protestant (76.19%) believe legal abortion can or should be allowed. 12.5% versus 76.19% is quite a big different." So you'd say, "It looks obvious that religion matters in the beliefs of legal abortion."
Now let's look at another table. I will show the percentage in the table -- the ratio between the opinions in a religious group.
religeon vs abortion opinion |
| Catholic | Protestant | other | total |
yes(%) | 18(45) | 25(62.5) | 12(60) | 55 |
no(%) | 22(55) | 15(37.5) | 8(40) | 45 |
Total(%) | 40(100) | 40(100) | 20(100) | 100 |
The situation is a bit different. Can you still say that there is a difference between the religions (the Catholic and the Protestant) in legal abortion? 45% of the Catholic and 62.5% of the Protestant agree with the idea of legal abortion. You might say, "Sure 45% and 62.5% are different." But, this does not seem right since the statement may mean that there should be the exact same percentage in each category in order to claim that there is no difference between religious groups. We all know that it is not going to happen in reality. This may lead you to an idea that you need some kinds of methods that can be used to aid your decision. And these "some kinds of methods" are so called statistics (in addition to what you already know (the characteristics of samples)) -- for this particular example, the chi-square test.
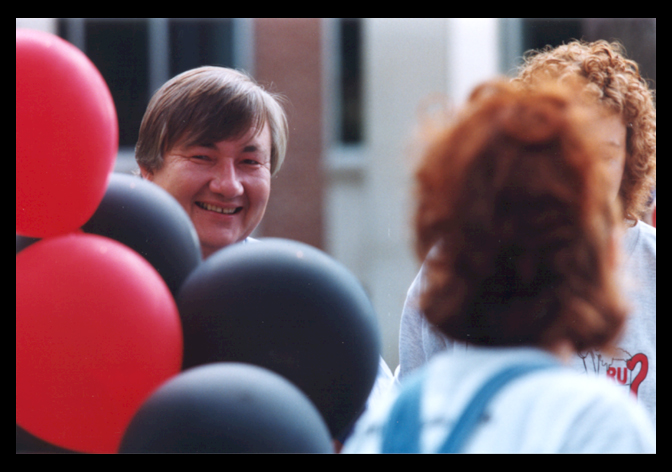
Dr Mokros at RuSure Campaign SCILS Rutgers 2000 [JPG image (202.12 KB)]
Chi-square test:: This is how the Chi-square method was involved in ... Anyway, now let's think about the chi-square test. Previously, you wanted to know if there are differences in the abortion opinions among religious groups; and you got the frequency table. Now think about what the table would look like if there is no differences in the abortion opinion among religious groups?
We already know from the first contingency, frequency, or bivariate analysis table that there were 50 people who were for legal abortion and 50 people who were against legal abortion -- the ratio of each category was (YES --> 50 out of 100; NO --> 50 out of 100). And there were 40 Catholics; 42 Protestant; and 18 others.
Chi-square table |
| | Religion |
| | Catholic | Protestant | Other | Total |
Legal Abortion | yes | | | | 50 |
| | . | | |
no | | | | 50 |
| | | . | | |
| Total | 40 | 42 | 18 | 100 |
If there is no difference, we can assume that the number of the Catholic (total 40) can be distributed according to the ratio of "yes" (50%) and "no" (50%) of the total. This might be a bit confusing... You may think of it in this way:
"In total, there were exactly half (50%) of the people who responded to the survey said yes. Therefore, if there would be no difference in the opinion within them, I can assume that the half of the Catholic -- half of the 40 -- would answer yes."
Lets think about another table, which I just made up:
religeon vs abortion opinion |
| Catholic | Protestant | Other | Total |
yes | | | | 60 |
Expected value | 24 | | | |
no | | | | 40 |
| 16 | | | |
Total | 40 | 42 | 18 | 100 |
Here, the ratios of yes and no are 60/100 and 40/100 -- 0.6 and 0.4.
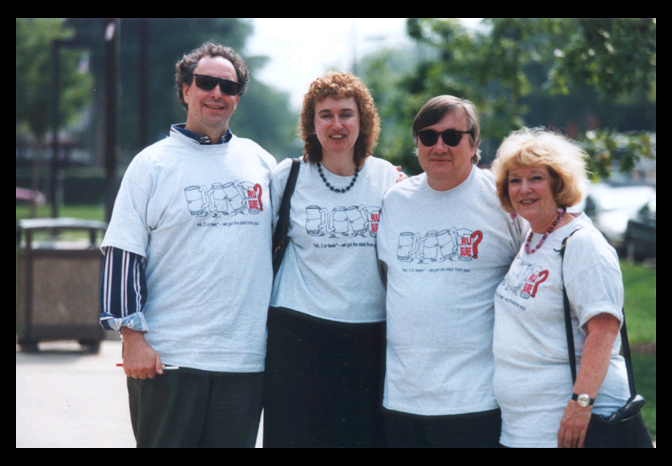
Professors at RUSURE campaign SCILS Rutgers 2000 [JPG image (611.69 KB)]
"60 out of 100 people (60/100; 0.6; 60%) said yes. Therefore, I can assume that the same ratio (60/100 = 0.6) of people in the Catholic would answer yes. 60% of 40 people is 0.6 x 40 = 24. By the same token, 40 out of 100 people (40/100; 0.4; 40%) said no. Therefore, I can assume that the same ratio (0.4) of people in the Catholic would answer no. 40% of 40 people is 0.4 x 40 = 16."
Let's get back to the original table. I wrote down the number of the
Yes cell. Remember this number represent your pure assumption about
no difference between or among the religious groups in the abortion issue.
religeon vs abortion opinion |
| Catholic | Protestant | Other | Total |
Yes | | | | 50 |
(Expected value) | 20 | | | |
No | | | | 50 |
(Expected value) | | | | |
Total | 40 | 42 | 18 | 100 |
Now, how about the Protestant? We also can assume that the same ratio (50 out of 100; 50/100; 50%) of the entire Protestant would say yes. The total number of the Protestant is 42. Therefore, the expected value (under the assumption of no differences among the religious groups) is 0.5 x 42 = 21. By the same way, you can assume that the half of the people in the "Other" category" would say yes -- 0.5 x 18 = 9. If you do the same thing over the entire cell in the table, the table would look like below.
religeon vs abortion opinion |
| Catholic | Protestant | Other | Total |
yes | | | | 50 |
Expected value | 20 | 21 | 9 | |
no | | | | 50 |
expected value | 20 | 21 | 9 | |
Total | 40 | 42 | 18 | 100 |
Let's put all the numbers in the same table now (see below).
Now you have two values for each cell. The numbers without parenthesis may be called
observed values -- you did count the number of the respondents. The numbers with parenthesis may be called
expected values -- they are expected to be those numbers
under the assumption that there is
no difference in "the abortion opinion" among the groups.
At this time, have a breathe and think about what you did. It -- obtaining the expected values -- is relatively simpler that you first thought. The idea was that if there is no difference among the group regarding the opinion about abortion, the ratio of "yes" and "no" in each religious group should be the same as that of the total group -- In other words, the ratio of "yes and no" (attributes of the dependent variable) in the total people should be applied to the each category of the independent variable.
religeon vs abortion opinion |
| Catholic | Protestant | Other | Total |
yes | 5 | 32 | 13 | 50 |
Expected value | (20) | (21) | (9) | 50 |
no | 35 | 10 | 5 | 50 |
| (20) | (21) | (9) | 50 |
Total | 40 | 42 | 18 | 100 |
Now the other part is examining the numbers. Do you remember you obtained the expected values because ... you wanted know what the table would look like if there is no differences in the abortion opinion among the religious groups? In other words, the expected values are ideal numbers for your testing idea -- religious groups are indifferent in abortion opinions. So you may have a rough idea that if the two numbers in a cell are approximately same, the chances are that you can say the observed sample indeed represents the indifference.
Let's think about samples -- keep your assumption that religious groups have no different ideas on legal abortion. Under this assumption, you obtained ideal types of numbers for the cells and called them expected values. And you expected that the numbers you get from your actual samples -- the observed values -- are the same as the expected values. But, you know that in reality they are going to be different.
If they are slightly different, you can say that the differences (between the observed values and the expected values) are purely
from some sorts of errors that randomly occurred in your sampling procedure -- you will see this phrase again later. If the differences are big enough, you can say that the religious groups are indeed different from each other regarding the legal abortion opinion. But, where is the line between these two -- a slight and a big difference?
Chi-square is the method to aid your decision about this. The procedure to obtain the chi-square is:
where,
O is observed value
E is expected value. Sometimes, it is called theoretical value. <
So, T is used instead of E.
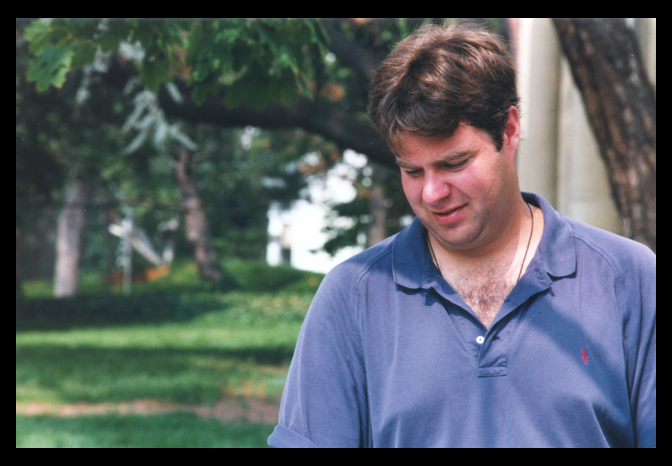
Brad Crownover (Comm Dep advisor) at RuSURE campaign SCILS Rutgers 2000 [JPG image (248.02 KB)]
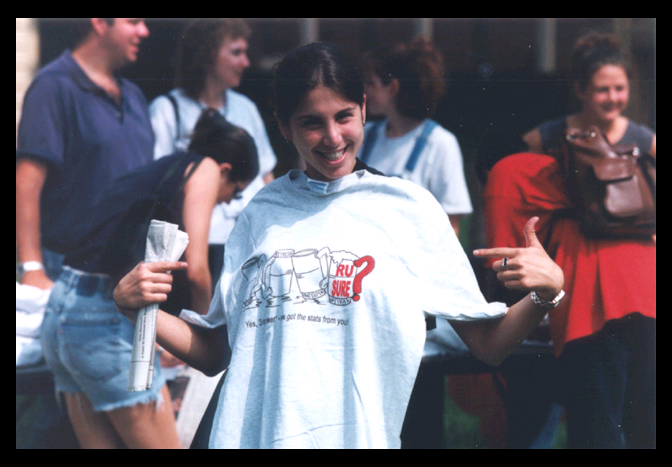
RUSURE campaign SCILS Rutgers 2000 [JPG image (613 KB)]
You may want to look up your textbook about this. The sign

is called Sigma and means that you sum up all the values involved in the calculation. In your example, you have 6 different cells and each cell has two values (expected and observed). You obtain the difference between the expected and observed value; square the result; and divide the result with the expected value. You keep this calculation for the other five cells and sum them up.
Let's see the table.
religeon vs abortion opinion |
| Catholic | Protestant | Other | Total | Chi-square |
yes | 5 | 32 | 13 | 50 | |
Expected value | (20) | (21) | (9) | 50 | |
(O-T)2 / T | (-15)^2^/20=11.25 | (11)^2^/20=5.76 | (4)^2^/20=1.78 | | 18.79 |
no | 35 | 10 | 5 | 50 | |
| (20) | (21) | (9) | 50 | |
| (15)^2^/20=11.25 | (-11)^2^/20=5.76 | (-4)^2^/20=1.78 | | 18.79 |
Total | 40 | 42 | 18 | 100 | 37.58 |
Chi-square value = 37.58.
Degrees of Freedom (df) = (the # of columns-1)x(the # of rows-1)= 2 x 1 = 2.
I do not know exactly why the degree of freedom is important in a conceptual way -- so, having a difficulty explaining it. But, the idea behind it is that if you know totals of column and row, and the values of two cells (as a minimum requirement; called degree of freedom), you would be able to obtain the values of the other four cells without consulting the actual observed values.
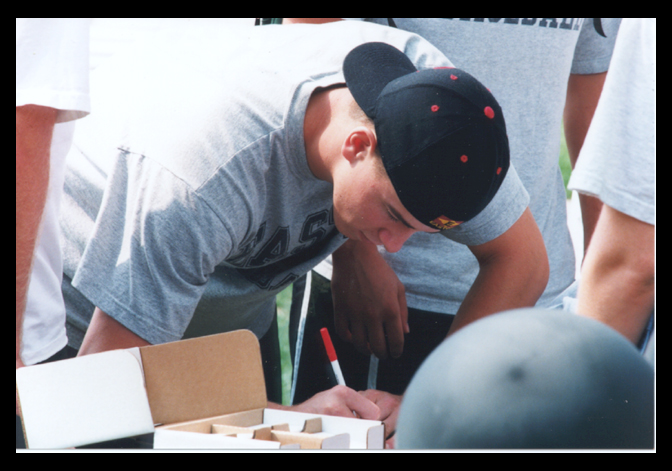
RUSURE campaign SCILS Rutgers 2000 [JPG image (218.12 KB)]
Anyway, you just obtained the chi-square value (37.58) and the degrees of freedom (2). You can look up the text book (for chi-square test): (1) find your degrees of freedom (2), that is, the second row of the table; (2) decide the probability you want to employ (usually .05 or .01); (3) write down the numbers; and (4) compare them to your chi-square value.
The numbers you obtain from the book are 5.991 for the 0.05 probability and 9.210 for the 0.01 probability. They are called critical values. So the critical values are:
5.991 at 0.05 probability
9.210 at 0.01 probability
These critical values do not exceed the chi-square value you obtained from your table -- 37.58. How do you want to relate them together? Think about the expected values -- the ideal types. Suppose you obtained the same values (observed values) as those of expected values, what would be your chi-square value? --Yes, it is going to be zero. Why? If you look at the formula
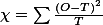
again, you see the difference between O and T is zero, since you assumed that the observed values (Os) are the same as those of expected values (Ts). Therefore, the values of (O - T) in
all cells are going to be zero. Therefore, the chi-square value is going to be zero.
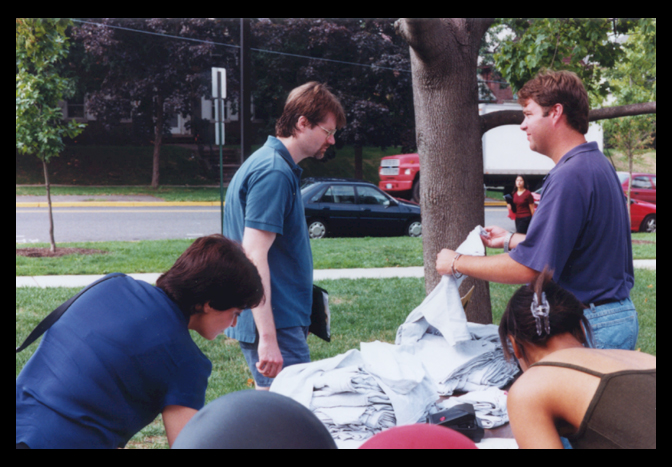
Dr. Mark Frank (Your favorate professor
.png)
) at RUSURE campaign SCILS Rutgers 2000
[JPG image (269.88 KB)]
Hence, if chi-square value you obtained is smaller than the critical value -- approaching zero in theoretically --, you can say that the difference of the two values (critical value and your chi-square value) is due to some variation that is randomly occurred in your sampling procedure. But, since the difference is big, that is, exceeding the critical value, you can safely argue that the difference is not due to the some sorts of random variation, but due to the fact that they are really different. What has this decision got to do with the level of probability (0.05 and 0.01)? Choosing probability means you accept or avoid your responsibility of stating the truth. That is, if you chose 0.05 probability, you are saying that your statement about the difference of the religious groups in the legal abortion opinion is true with 0.95 out of 1 certainty. That is, your chi-square number
that seems different enough from the critical value to say that the religious groups have different ideas about legal abortion may be due to the nature of the some sorts of errors that randomly occurred in your sampling procedure. However, the chances that this is going to happen is about 0.05 out of 1 (5 out of 100 cases; 5%). So, basically, choosing the probability means you decide the certainty of your decision. At the same token, since the chi-square value is even bigger than the critical value at 0.01 probability level, you can state that there is indeed difference in legal abortion opinions among the religious groups. The chances that you are wrong about this decision is 0.01 out of 1 (1 out of 100; 1%). That is, even though the difference of the chi-square and the critical value seem to be due to the fact that the religious groups are indeed different in legal abortion issue, there is still a slight chance of error -- such a difference between the chi-square and critical value is due to the randomly occurred error in your sampling procedure. And such chances are 1 out of 100.
And this "chance of making an error in your decision" is called what? --> alpha.
Let's have an exercise next.
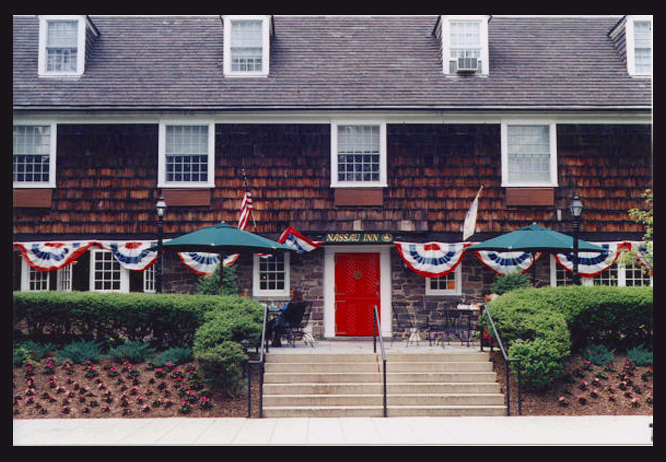
Naussau Inn near Princeton University [JPG image (601.03 KB)]
- Chi-square test, example
- Let's have an exercise for the chi-square thing. I hope you remember the below part which is from the last essay I wrote. Let's go through it, first. Let's look at another table. I will show the percentage in the table -- the ratio between the opinions in a religious group.
Abortion opinion and Religeon |
| Catholic | Protestant | other | Total |
yes | 18 | 25 | 12 | 55 |
% | (45) | (62.5) | (60) | |
no | 22 | 15 | 8 | 45 |
% | (55) | (37.5) | (40) | |
| 40 | 40 | 20 | 100 |
The situation is a bit different. Can you still say that there is a difference between the religions (the atholic and the Protestant) in legal abortion? 45% of the Catholic and 62.5% of the Protestant agree with the idea of legal abortion.
You might say, "Sure 45% and 62.5% are different." But, this does not seem right (even it looks ridiculous) since the statement may mean that there should be the exact same percentage in each category (say, 50% versus 50%). We all know the reality is not like that.
This may lead you to an idea that you may need some kinds of methods that can be used to aid your decision. And these "some kinds of methods" are so called statistics. Note the sentence, "Sure 45% and 62.5% are different." And let's see if it turnes out to be right. The below is the table with raw numbers -- observed values. I would like to ask you to fill out the expected value under each observed value -- total 6 cells.
Abortion opinion and Religeon |
| Catholic | Protestant | other | total |
yes | 18 | 25 | 12 | 55 |
expected value | a | b | c | |
no | 22 | 15 | 8 | 45 |
expected value | x | y | z | |
| 40 | 40 | 20 | 100 |
What did you get? Let's go through the first,
YES, cell. We need to figure out the ratio of yes and no
attributes (or values) of the dependent variable of the total samples. That is 55 out of 100 for the "yes" attribute; and 45 out of 100 for the "no" attribute -- 0.55 vs. 0.45. Remember that expected values are obtained based on the assumption of the no difference among the groups in the abortion opinions? Then, the expected value for the first cell -- that is, that we make up the number under the assumption of "no difference among the religious groups" -- would be
the same ratio of the "yes" attribute of the total samples -- 55/100 out of the total number of the Catholic. So, 0.55 X 40 = 22.
How about
c cell? We have total 20 people in "other" category (an attribute of the independent variable). So, 0.55 X 20 = 11. Lastly, how about
y cell? In this case, we are talking about "no" attribute; and the ratio of the "no" attribute of the total sample was 45/100. The total number of the Protestant is 40 -- note that it is not the number of the Catholic. So the expected value for the cell,
y, is 0.45 X 40 = 18.
*** Note: Hey! the calculated expected values are all whole numbers! Guess how much time I spent in making up the example table! My point is that you might not get the whole numbers
such as 1, 2, 3, 4, ... as your expected values in your table. Anyway, can you fill the entire cells now? It should look like the below.
Abortion opinion and Religeon |
| Catholic | Protestant | other | total |
yes | 18 | 25 | 12 | 55 |
expected value..... | (22) | (22) | (11) | (55) |
no | 22 | 15 | 8 | 45 |
expected value..... | (18) | (18) | (9) | (45) |
| 40 | 40 | 20 | 100 |
Now calculate the chi-square value. For the reference, the formula was as follows.
where,
O is observed value
E is expected value. Sometimes, it is called theoretical value (T).
abortion opinion and religion |
| Catholic | Protestant | Other | Total | Chi-square |
yes | 18 | 25 | 12 | 55 | |
Expected Value | (22) | (22) | (11) | (55) | |
(O-T)2 / T | (-4)2/22=0.73 | (3)2/22=0.41 | (1)2/11=0.09 | | 1.23 |
no | 22 | 15 | 8 | 45 | |
Expected Value | (18) | (18) | (9) | (45) | |
(O-T)2 / T | (4)2/18=0.89 | (-3)2/18=0.5 | (-1)2/9=0.11 | | 1.5 |
Total | 40 | 40 | 20 | 100 | 2.73 |
Chi-square value = The sum of the entire 6 yellow cells = 2.73.
Degrees of Freedom (df) = (the # of columns-1) x (the # of rows-1)= (3-1) x (2-1) = 2 x 1 = 2.
Look up the values in your textbook -- which is called "critical values."
They are:
5.991 (0.05 probability)
9.210 (0.01 probability)
Now the rest of what you need to do is to compare the numbers (chi-square value and the critical values).
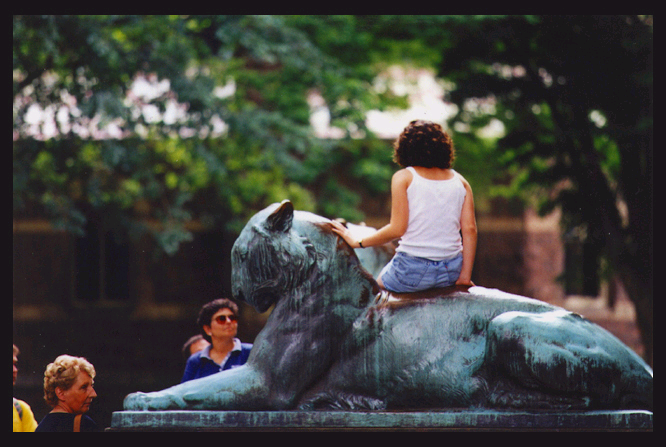
Princeton University Tour [JPG image (581.51 KB)]
The chi-square value is . . . smaller than the critical values! At this point, you should not think or say "So what?"! If you are confused with the numbers, think the basics first. That is, if the observed values are the same as the expected values, the calculated chi-square would be zero. So, if there is going to be differences among the cells
differences in abortion opinions among the religious groups, the chi-square value should be big -- the difference between the observed value and expected value for each cell should be big.
The result was that the chi-square value was SMALLER than the critical value for 0.05 probability as well as for the 0.01 probability. Therefore, you can state that there seems no differences in the abortion opinion among the religious groups. Sounds like we skip something -- hard to relate what you found as chi-square to the conclusion?
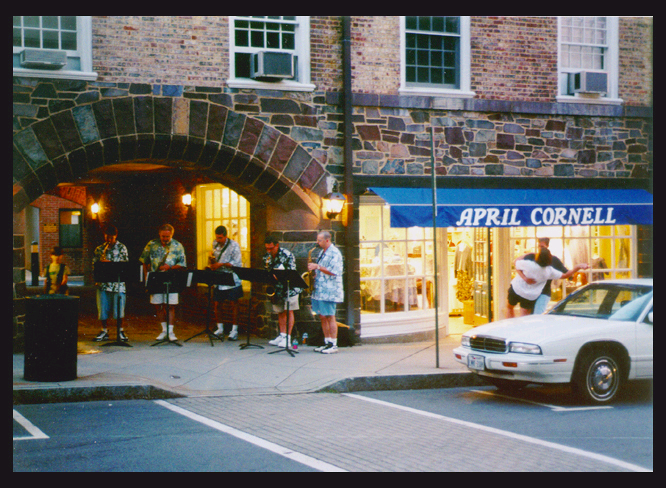
Princeton University Mall on Friday [JPG image (634.85 KB)]
In the first place, you assumed that there would be no differences in the abortion issue among the religious groups to get the expected values. And you compared the expected values to the observed values. In other words, you tested your survey result (the observed values) against the idea of "no difference." Your plans were: If the some of the comparison (chi-square) is big enough, you'd say that the idea of "no difference" was not likely true. If the some of the comparison (chi-square) is small enough, you'd say that there seems to be no reason to reject the idea of "no difference." In other words, in the first place, you assumed that there would be no difference, and you tested your survey result against this idea. What you conclude from this testing was you failed to disapprove the idea -- the idea of no differences.
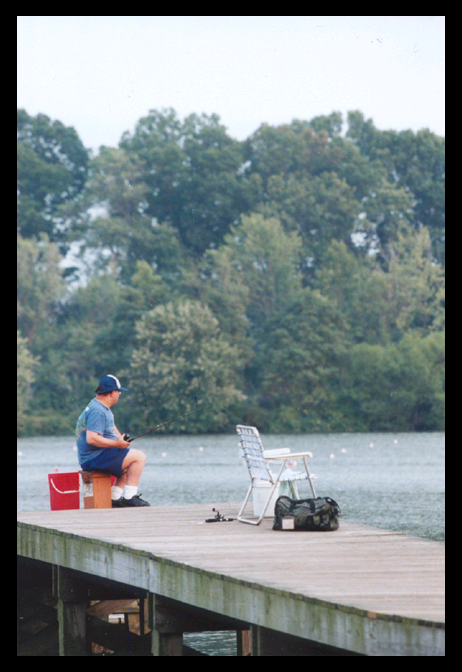
Princeton Park river [JPG image (606.44 KB)]
*** Why null? -- Someone in the class cleverly asked why we should use null hypothesis in the first place. As you see the above, it would be harder to test whether the researcher is right. Most statistic methods (chi-square, t-test, ANOVA, and others) test against the idea of 0 (zero -- no difference).
Therefore, it would not have been safe, had you ever said, "Sure 45% and 62.5% are different."
*** Another note: You might have a question... Hey, wait a minute... If I pick up some other numbers from the chi-square distribution table, the result would be totally different!
*** For your information, the table looks as follows. And the chi-square value you got from your data was 2.73.
df | .30 | .20 | .10 | .05 | .02 | .01 | .001 |
1 | 1.074 | 1.642 | 2.706 | 3.841 | 5.412 | 6.635 | 10.827 |
2 | 2.408 | 3.219 | 4.605 | 5.991 | 7.824 | 9.210 | 13.815 |
... | ... | ... | ... | ... | ... | ... | ... |
... | ... | ... | ... | ... | ... | ... | ... |
If you want to pick up a different probability -- rather than 0.05 or 0.01 whose critical values are 5.991 and .210 respectively, you may have something different conclusion. But, they are not going to be the probability of 0.10 or even 0.20 because the critical value for each probability is still bigger than your calculated chi-square value (2.73). You may pick the far left column -- the critical value in the first column -- 0.30 probability -- is smaller! And you may say that there are differences in abortion opinions among the religious groups! Sure you can. But, think about the responsibility that you are taking with the statement. How much responsibility you are taking now for saying that? Let's go back to what I said earlier.
Choosing probability means you accept or avoid your responsibility of stating the truth. That is, if you chose 0.05 probability, you are saying that your statement about the difference of the religious groups in the legal abortion opinion is true with 0.95 out of 1 certainty. That is, your chi-square number
that seems different enough from the critical value to say that the religious groups have different ideas about legal abortion may be due to the nature of the some sorts of errors that randomly occurred in your sampling procedure. However, the chances that this is going to happen is about 0.05 out of 1 (5 out of 100 cases; 5%).
So, basically, choosing the probability means you decide the certainty of your decision.
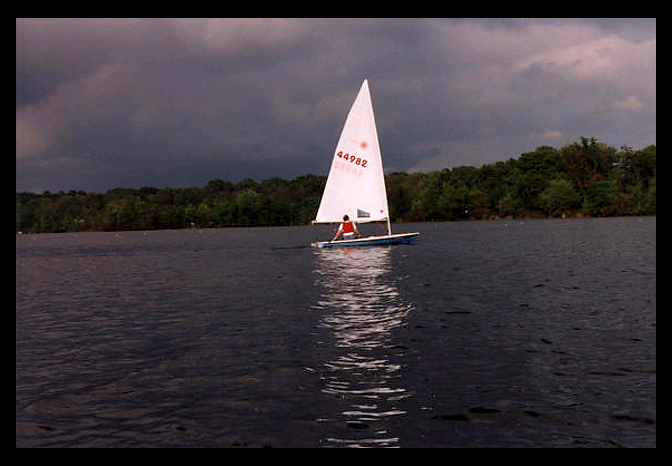
Princeton Park river [JPG image (611.69 KB)]
At the same token, since the chi-square value is even bigger than the critical value at 0.01 probability level, you can state that there is indeed difference in legal abortion opinions among the religious groups. The chances that you are wrong about this decision is 0.01 out of 1 (1 out of 100; 1%). That is, even though the difference of the chi-square and the critical value seem to be due to the fact that the religious groups are indeed different in legal abortion issue, there is still a slight chance saying that such a big difference between the chi-square and critical value is due to the randomly occurred error in your sampling procedure. And such chances are 1 out of 100.
Yes, choosing the probability means that you decide the certainty of your decision. The chances of your being wrong in your statement -- there is differences in the abortion issue among the religious groups -- was 3 out 10. And unlike the probability of 0.05 or 0.01, this risk is too big to take. In other words, it is a bit meaningless. This is why professor White said that social scientists usually take 0.05 as a criterion of his or her statistical tests.